A virtual simulator teaches autonomous cars to see and understand cities
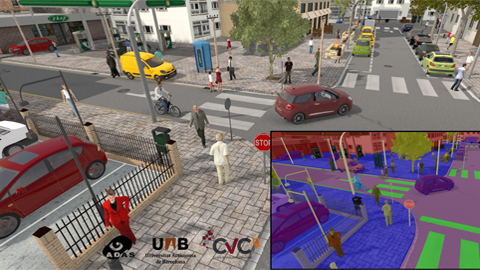
17/06/2016
The project developed by the Computer Vision Centre is led by researcher Germán Ros and Antonio M. López, who is also lecturer of the Department of Computer Science.
At the moment, self-driving cars such as the Google car or Tesla vehicles need to develop a “basic intelligence” which allows them visually to identify and recognise different elements such as roads, pavements, buildings, pedestrians, bicyclists, etc. In the end, these cars must see and understand roads exactly as we humans do.
“These vehicles require the use of artificial intelligences (AIs) to understand what is happening in their surroundings and depend on artificial systems which simulate the functioning of human neural connections. Our simulator, SYNTHIA, represents a giant leap within this process”, Germán Ros says.
SYNTHIA is capable of accelerating and improving the way in which artificial intelligences learn how to understand a city and its different elements. That represents a crucial advance in one of the greatest challenges in the field of self-driving cars: showing the car to identify what is happening around it. The data generated by the simulator will be shared openly and freely with the scientific community at the end of June in Las Vegas, in the International Conference on Computer Vision and Pattern Recognition, one of the most prestigious of its sector. By making the information available, scientists hope that advances will be made in the area of artificial intelligence and autonomous cars.
Until now, the main limitation in the development of artificial intelligences has been the large volume of data and human effort required to make them learn complex visual concepts in diverse conditions (e.g., the difference between the pavement and the road on a very rainy day). A tedious and highly expensive process which could require thousands of hours of constant supervision by human operators.
SYNTHIA is presented as a new technology which uses a virtual simulator to generate artificial intelligences in a simple manner and without the need of human interventions. The simulator is capable of generating all the additional information needed to supervise the AIs learning process in a fully automated manner, with no need for human interventions. Thanks to this, there are no more limitations due to the need for human operators (who can also make mistakes) and there is a drastic drop in costs related to producing intelligence agents, making it possible to develop more sophisticated and safer self-driving systems.