Seminar Paula de la Lama
In Seminar Room E2 at 15:00h
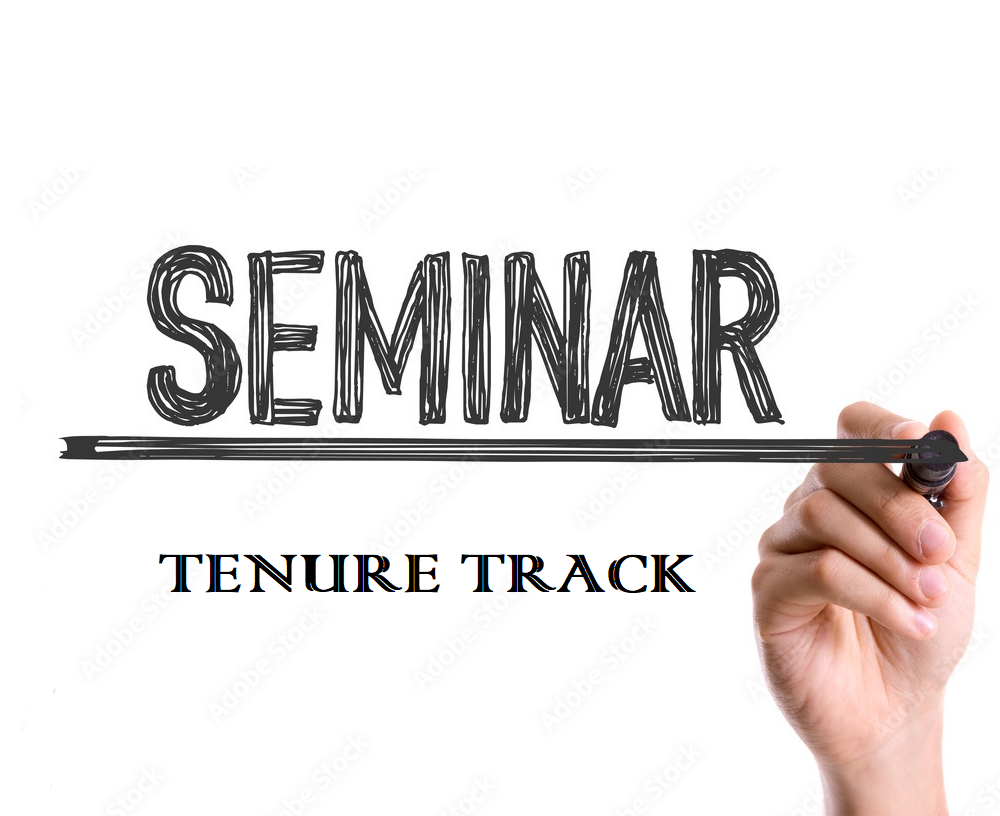
Paula de la Lama (Universitat de Girona) will present her paper “A compositional optimization for a convex clustering method”
The invited speaker is interested in a job tenure track in the department (Area: Operations)
Abstract:
This study introduces an innovative clustering approach, specifically designed for convex Qmode clustering (grouping by variables) within the Simplex space employing Aitchison geometry. The Simplex, derived from compositional data (CoDa), represents datasets where observations refer to relative parts of a whole, with a constant total sum of the parts. These datasets often require specialized analytical methods for accurate interpretation.
Our method is crafted to generate more coherent and interpretable clusters. Harnessing an operator splitting solver for quadratic programs (OSQP), we employ a convex relaxing hierarchical clustering technique. The objective function is formulated utilizing three distinct approaches of the L^1_CoDa-norm. The L^1-plr norm, the L^1-clr norm, and the Aitchison norm (L^2 ). The efficacy of this methodology is demonstrated through the analysis of paleontological and geochemical data, highlighting its potential to reveal meaningful patterns within complex and resilient datasets.